In order to make sustainable agriculture accessible globally, we need to access and build upon industry-leading science and technology. This science and tech ensures that we have strong, verifiable data to back up our sustainability claims, get farmers the support they need and help leaders build a business case for sustainable farming.
Let’s take a deep dive into the science we use to achieve our goals.
What parts of sustainable agriculture can we assess?
- GHG emissions: what types of greenhouse gasses are emitted, and in what quantity, as a result of food production?
- Soil Carbon: how much existing carbon can we pull from the atmosphere and sequester in agricultural soil?
- Food resilience: how can our crops be strong and productive in the face of our changing climate?
- Ecosystem services: how can we minimize land use and maintain biodiversity with agriculture?
- Air quality: how can we ensure good air quality in our agricultural systems?
- Water quality: how can we ensure that our farming efforts don’t negatively affect the water in our environment?
It’s essential that we accurately measure the outcomes of sustainable farming practices. Without the data, how could anyone know whether those efforts are making a difference? We also need to be able to estimate or model future emissions based on the adoption of different regenerative farming practices.
“We are not only at the frontier of building new products, but we’re also at the frontier of scientific knowledge. We are both creating new knowledge, and we’re taking the existing knowledge we have, building new methods with it and scaling it.” — Gayathri Gopalakrishnan, Ph.D., Director of Environmental Science
Modeling agricultural systems is complicated
Because agriculture itself is complicated.
Agricultural emissions vary on a field-by-field basis due to the crop produced, the composition of the soil in the field, weather conditions in a specific region, agricultural management practices, and many other factors.
At Regrow, we use an environmental model called DeNitrification DeComposition, or DNDC to model agricultural systems. DNDC takes data about emissions and carbon sequestration (how much carbon is stored in the soil) for agricultural systems, and uses that data to predict how emissions may change as producers adjust their farming practices.
Regrow’s data accounts for nuanced systems.
Emissions factors are often calculated using generalized datasets, and carbon sequestration is often measured with soil sampling. Both of these methods have challenges associated with them.
Generalized databases provide a good starting point for quantifying emissions, but these datasets don’t account for the variation between different farming systems in a given region. For example, they fail to address how emissions change if the same crop is grown in different counties within the same state, or in a different type of soil.
Regrow’s model is useful because it is dynamic — it builds upon new datasets, and has the potential to expand in its estimation capabilities.
Soil sampling provides an accurate account of the carbon stored in agricultural soils, but it’s an inefficient process.
With the right model (like Regrow’s), we can predict the emissions and sequestration associated with crop production without spending too much time and money on soil sampling.
As an example, here’s a simplified diagram of the calculation factors in the DNDC model:
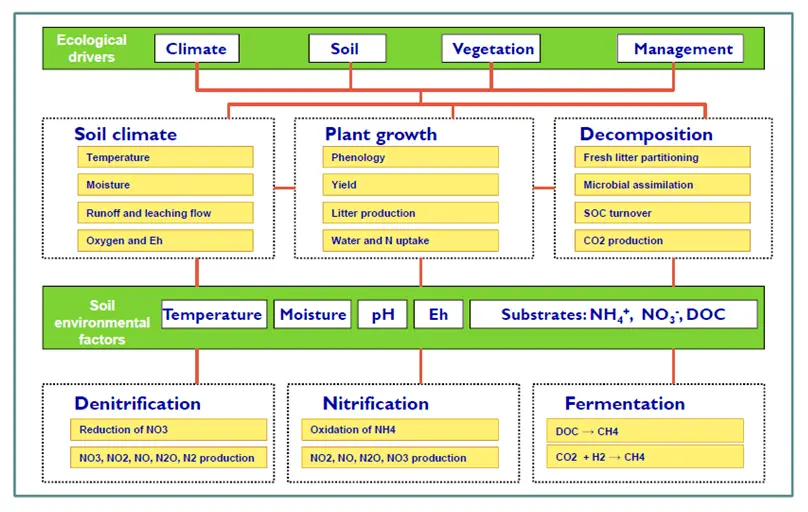
We calibrate our model diligently.
In order to build upon the DNDC model and ensure the most accurate outcomes possible, our team asks a series of questions:
- What are the processes governing these systems that we know about? Can we write equations that represent these processes?
- What data are needed for these processes? Can we collect the data? If so, how accurate is the data?
- What results can we get from this data? Are the results reproducible? Can we compare them against observed data, such as soil samples and satellite data?
Our soil nutrient cycling model, DNDC, has stored data from numerous agricultural systems, and is using that data to predict emissions and sequestration levels for crop production. However, our team is always working to enhance this model, and to expand DNDC’s capabilities through the input and analysis of new data.
We constantly test our data and its sources.
As we work to expand DNDC and incorporate this environmental model into Regrow’s products, we are constantly testing the validity of the model and the data behind it. In expanding DNDC, we always consider the following factors:
- Where our data comes from (In-field measurements, remote sensing algorithms like OpTIS, farm management systems (FMS), government databases such as the National Weather Service and the Natural Resources Conservation Service, etc.)
- What assumptions we will need to make, if data is not available
- What impact our assumptions will have on the accuracy and reliability of our model
These considerations ensure that we expand our knowledge and capabilities in an ethical, scientifically rigorous way, and that the estimates produced by our model will contribute to a healthier, greener planet.
We are committed to scaling our impact.
The scientific process is essential to our work at Regrow. Our teams are constantly working to run our model, test its predicted outcomes against field observation data, and get the best results possible. Once we’ve filled the gaps in our knowledge, it’s time to find new gaps, access new data and scale our work.
This is an ongoing process not only with our soil nutrient cycling model, but also with our monitoring technology and the products we deliver to our customers. Careful research and scientific rigor are at the heart of our mission: to make sustainable agriculture accessible on a global scale.